Sacramento River Basin serves as a test case for conserving water over unpredictable weather cycles
The summer of 2022 brought worrying news for California’s water supply.
Shasta Lake (seen above in 2024, having filled back up), the state’s largest reservoir and a critical source of water for millions, saw its levels drop to 38% of capacity in July — a historic low for that time of year. That drought laid bare a growing challenge: how to balance immediate water demand with long-term supply in an era of increasing climate uncertainty.
In a working paper, UCLA Anderson’s Felipe Caro, University of Mannheim’s Martin Glanzer and UCLA Anderson’s Kumar Rajaram develop a model for the management of reservoir systems over the long term. It’s designed to minimize societal costs of a water shortage. In a case study of California’s Sacramento River Basin, the authors’ management policy reduced average shortage costs — the cost of getting water from other, last-resort, sources — by 40% compared with the current policy, potentially remarkable savings.
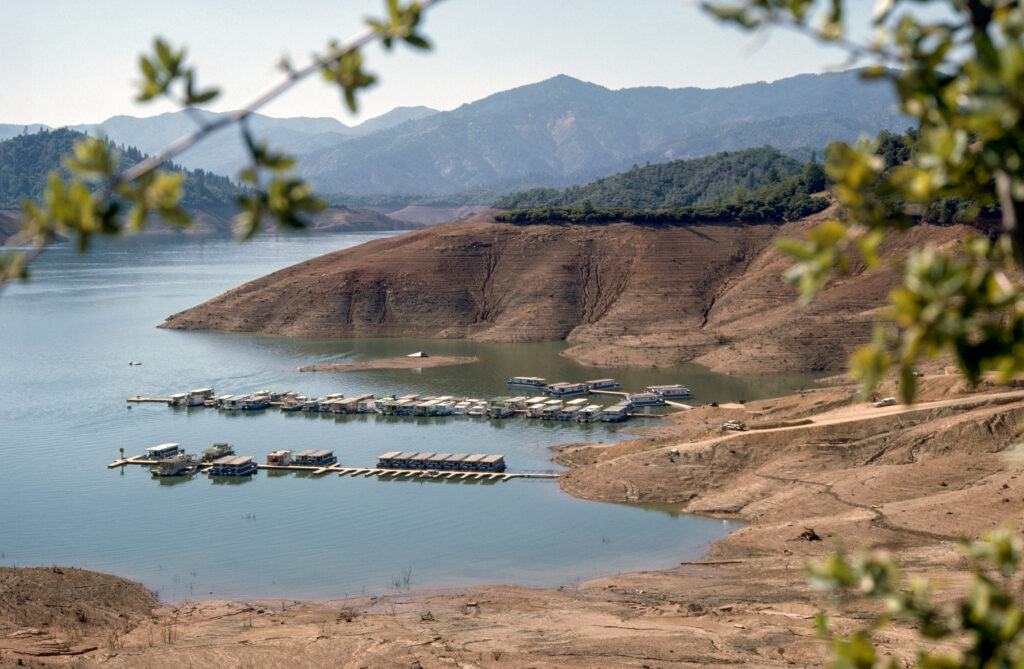
Drought left Shasta Lake at 28% of its total capacity in September 1976.
Thinking in Cycles, Not Years
Instead of the fixed or infinite timelines used by most traditional models, the authors’ approach uses cycles. A cycle begins when all reservoirs in a system like the Sacramento River Basin are simultaneously full and ends when they reach that state again — regardless of how many years it takes. Sacramento River Basin’s last complete cycle stretched from 2006 to 2019.
California’s reservoirs can be thought of like a network of interconnected buckets — each varying in size and refilled by unpredictable amounts of rainfall and snowmelt. Humans can determine when to empty the buckets, but only Mother Nature determines the timing to refill them. When trying to make the best decisions to manage these water resources, traditional models face three key problems:
- Short-term planning risks depleting reservoirs too quickly during dry years.
- Fixed-time horizons force artificial deadlines, leading to short-sighted decisions — one cycle may be considerably longer than the last.
- Long-term models struggle to accurately value future water needs, often favoring short-term use and risking long-term shortages.
The study focuses on three major reservoirs in the Sacramento River Basin, each with unique characteristics: Shasta Lake (slow to fill, large capacity), Trinity Lake (moderate filling rate) and Folsom Lake (the smallest of the three, quick to fill). The researchers used publicly available data from the California Data Exchange Center covering the period from May 1, 2000, to April 30, 2024. The data included daily inflow measurements, evaporation rates and reservoir capacities. May marks the beginning of the dry season, so the data enables the researchers to analyze 24 complete seasonal changes.
Rather than treating each of the three reservoirs separately, the researchers’ model first combines them into one “virtual reservoir” to determine optimal total water releases. This aggregation allows the researchers to overcome the “curse of dimensionality” — the exponential increase in computational complexity when managing multiple factors (reservoirs in this case).
To handle climate uncertainty, the researchers developed a framework that prepares for the unexpected. Instead of relying solely on historical weather patterns, which may become less reliable due to climate change, the model assumes nature acts as an adversary, always choosing the worst-case scenario for water inflow. However, the model prevents overreaction with a system that quantifies how much a predicted weather scenario deviates from expected patterns.
Caro, Glanzer and Rajaram’s approach seeks to keep the model cautious without becoming overly conservative. When extreme scenarios are predicted, the system forces the model to weigh them against more typical patterns, avoiding under- and overreacting. The model then allocates water releases based on each reservoir’s unique characteristics. For example, Folsom Lake might release a larger portion of its water compared with Shasta Lake because Folsom can refill more quickly.
Testing with real data revealed the following observations:
- A 40% reduction in average water shortage costs compared with current practices.
- Performance within 3% to 15% of the mathematically best possible solution.
- Higher overall storage rates across all reservoirs.
- Better performance during critical drought periods.
As can be seen in the charts below, the current management policy led Trinity and Folsom lakes to reach their minimum levels during the 2015 drought. The researchers’ model maintained higher water levels, by better anticipating and preparing for the drought conditions.
The model is more than simply hoarding water. It finds a strategic balance — when it withholds water, it’s in ways that avoid causing harm. In wet years, traditional models tend to release too much, missing a chance to rebalance storage across reservoirs. This model uses those opportunities to prepare for future dry periods by doing such rebalancing. It also prioritizes essential uses like drinking water, while delaying nonessential uses — like irrigation for certain crops — when future supply looks uncertain.
The implications of the researchers’ work extend beyond California. As climate change increases weather volatility worldwide, this cycle-based approach could help water managers make decisions about when to store and when to release water. The model’s optimization framework could make it particularly valuable in regions facing increasing climate uncertainty.
Caro, Glanzer and Rajaram’s work suggests that by working with nature’s cycles rather than against them, we can dramatically improve how we manage our water resources.
Featured Faculty
-
Felipe Caro
Professor of Decisions, Operations and Technology Management
-
Kumar Rajaram
Professor of Decisions, Operations and Technology Management
William E. Leonhard Chair in Management
About the Research
Caro, F., Glanzer, M., & Rajaram, K. (2024). Sustainable Management of a Water Reservoir System Facing Climate Uncertainty. Available at SSRN 5008922.